In today’s competitive business world, understanding which potential customers, or leads, are most likely to make a purchase is crucial. This is where lead scoring comes in. Lead scoring is a method used to rank leads based on their potential value to the company. It helps sales teams focus their efforts on the most promising leads, saving time and improving efficiency.
Earlier, manual lead scoring has been the traditional way to do this. It involves assigning points to leads based on certain criteria, such as their engagement with the company’s website or their responses to emails. However, this method can be time-consuming and sometimes inaccurate.
The advent of predictive analytics has made things a lot smoother and easier. This technology uses data and statistical methods to predict future outcomes. In the context of lead scoring, it means using data to predict which leads are most likely to convert into customers. This process, known as predictive lead scoring, is becoming increasingly popular.
Predictive lead scoring models analyze a vast amount of data to determine the likelihood of a lead converting. This not only speeds up the lead scoring process but also increases its accuracy. As a result, sales teams can prioritize their efforts more effectively, and marketing teams can tailor their strategies to target the right audience.
The purpose of this blog is to explain what predictive lead scoring is and why it matters for your business. We will explore the predictive lead scoring models, the benefits of using them, and how they can enhance your sales and marketing efforts. We will also discuss the steps for implementing predictive lead scoring and the impact it can have on your overall business strategy.
Lead Scoring Predictive Analytics
Definition of Predictive Lead Scoring
Predictive lead scoring uses data and machine learning to forecast which leads are most likely to become paying customers. It involves analyzing various data points to predict lead behavior and assigning scores accordingly.
Explanation of Predictive Lead Scoring
- Data Analysis: Predictive lead scoring systems analyze large datasets, including past customer behavior and engagement metrics.
- Machine Learning Models: These systems use machine learning algorithms to identify patterns and predict future outcomes.
- Automation: By automating the scoring model, predictive lead scoring helps reduce human error and improve accuracy.
- Continuous Improvement: The models continuously learn and improve over time, becoming more accurate as more data is processed.
Differences Between Traditional and Predictive Lead Scoring
Data Handling:
- Traditional Scoring: Relies on manual scoring methods where human input is necessary to assign points based on predefined criteria.
- Predictive Lead Scoring: Utilizes AI predictive lead scoring to analyze large datasets automatically, making it more efficient.
Accuracy:
- Traditional Scoring: Susceptible to human error due to manual data entry and subjective judgments.
- Predictive Lead Scoring: Offers higher accuracy by using data-driven insights and eliminating human bias.
Efficiency:
- Traditional Scoring: Time-consuming and requires significant human resources.
- Predictive Lead Scoring: Streamlines the sales process by quickly identifying the most promising prospects, enhancing overall marketing and sales efforts.
Adaptability:
- Traditional Scoring: Static and inflexible, often failing to adapt to changing market conditions.
- Predictive Lead Scoring: Dynamic and continuously evolving as the scoring model learns from new data.
Outcome:
- Traditional Scoring: Often identifies a qualified lead based on a limited set of criteria.
- Predictive Lead Scoring: Provides a more comprehensive and accurate prediction of lead quality, significantly improving the chances of conversion.
Importance in Modern Sales Strategies
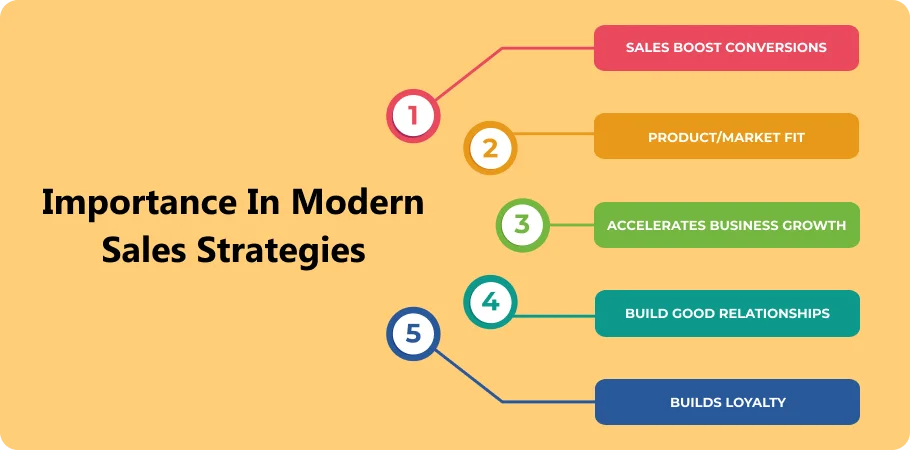
Enhanced Sales Strategies:
Predictive lead scoring helps enhance sales strategies by providing deeper insights into lead behavior.
It enables sales teams to focus on the most promising prospects, increasing the likelihood of conversion.
Value to Sales and Marketing Teams:
AI predictive lead scoring reduces time spent on manual scoring, allowing teams to focus on strategic tasks.
It helps prioritize leads more effectively, ensuring that both sales and marketing teams target leads with the highest potential.
Improved Collaboration:
Predictive lead scoring fosters better collaboration between sales and marketing efforts by aligning both teams with data-driven insights.
It ensures that marketing campaigns are tailored to attract high-quality leads, while sales efforts are concentrated on leads most likely to convert into paying customers.
In summary, predictive lead scoring is a powerful tool that leverages machine learning to improve lead scoring accuracy and efficiency. It plays a vital role in modern sales strategies, helping businesses identify and prioritize leads more effectively, thereby enhancing overall marketing and sales efforts.
How Predictive Analytics Works
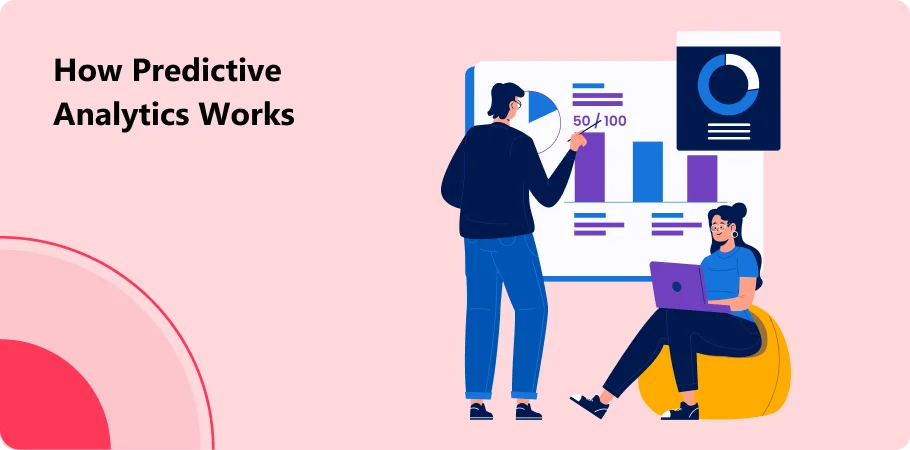
Data Collection: Predictive lead scoring works by gathering all the data related to potential customers. This includes past behaviors, demographic information, and interactions with the company.
Data Cleaning and Preparation: The collected data is then cleaned and organized to ensure accuracy and consistency.
Model Building: Using machine learning, a lead scoring model is created. This model analyzes patterns in the data to predict future behaviors.
Scoring and Analysis: The model assigns scores to leads based on their likelihood to convert. These scores help the sales team prioritize leads.
Key Algorithms and Techniques Used in Predictive Lead Scoring
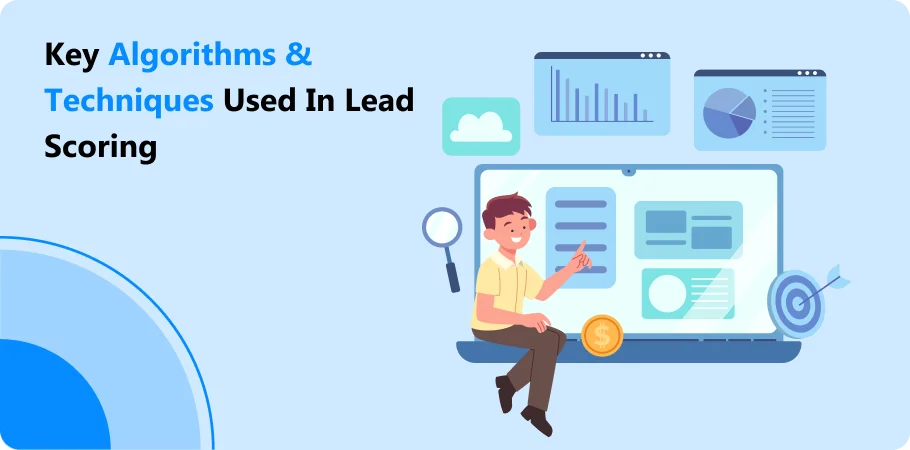
Regression Analysis: This technique examines the relationship between variables to predict outcomes.
Classification Algorithms: These algorithms sort leads into categories based on their likelihood to convert.
Clustering: Clustering groups similar leads together, making it easier to identify high-potential leads.
Machine Learning: Machine learning algorithms continuously improve the lead scoring model by learning from new data.
Benefits of Using Predictive Analytics for Lead Scoring
Improved Accuracy in Lead Prioritization
Higher Precision: Predictive analytics provides a more precise way to prioritize leads. This reduces the chances of missing high-potential leads.
Data-Driven Decisions: The benefits of predictive lead scoring include making decisions based on data rather than intuition, leading to better outcomes.
Enhanced Ability to Forecast Lead Behavior and Sales Outcomes
Predictive Insights: Predictive lead scoring offers valuable insights into lead behavior, helping the sales team understand which leads are more likely to convert.
Better Planning: With accurate forecasts, marketing and sales teams can plan their strategies more effectively, improving overall sales and marketing strategies.
lead nurturing: Predictive lead scoring helps in lead nurturing by identifying the right leads to focus on, ensuring that marketing efforts are targeted and effective.
In conclusion, predictive analytics plays a crucial role in lead scoring by enhancing the accuracy and efficiency of the lead generation process. It helps marketing and sales teams make better decisions, prioritize leads effectively, and improve overall sales and marketing strategies.
Essential Elements of a Successful Lead Scoring Model
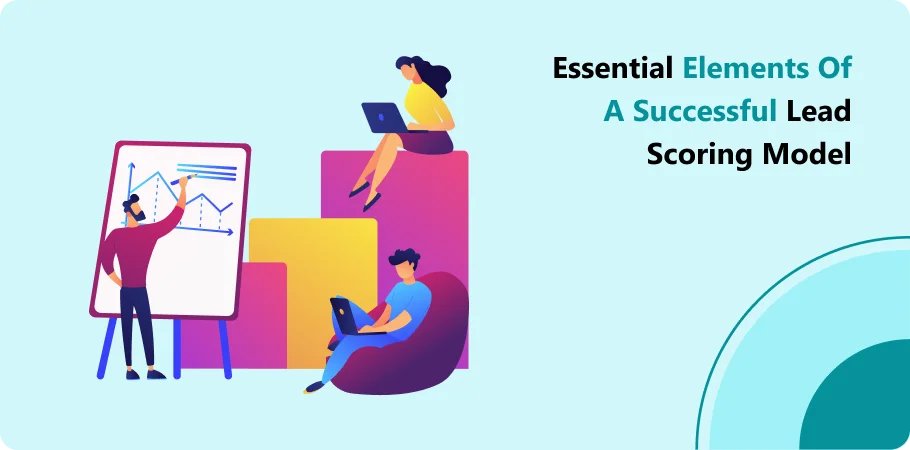
Criteria Definition: Clearly define what makes a good lead. This involves setting criteria that reflect the lead’s potential value.
Scoring Metrics: Develop a score based on various metrics such as engagement, behavioral data, and demographic information.
Algorithm Selection: Choose the right algorithms for your model. This could include regression, classification, or clustering techniques.
Regular Updates: Continuously update the model with new data to keep it accurate and relevant.
Factors Influencing Lead Scores
Behavioral Data: Actions taken by leads, such as website visits, email opens, and downloads. This helps predict a lead’s likelihood to convert.
Demographic Information: Data such as age, location, and job title. These factors help identify high value leads.
Past Data: Historical behaviors and past data provide insights into future actions and conversion rates.
Types of Data Used in Predictive Lead Scoring
Historical and Current Data: Both types of data are crucial. Historical data helps in understanding past behaviors, while current data shows the latest interactions.
Behavioral Data: Includes actions taken by leads like website visits and email interactions.
Demographic Data: Information about the lead’s background such as age, location, and industry.
Importance of Data Quality and Integrity
Accuracy: Ensuring data is accurate is vital. Poor quality data leads to incorrect scoring, wasting valuable sales resources.
Relevance: Use relevant data that directly impacts the lead scoring model. Irrelevant data can skew results.
Consistency: Consistent data collection and maintenance ensure the model remains effective over time.
Integrity: Maintain the integrity of data by regularly cleaning and updating it. This helps in making sure that the data is reliable and useful for scoring.
In summary, building an effective lead scoring model involves defining clear criteria, selecting the right metrics, and continuously updating the model with historical and current data. The marketing team must ensure data quality and integrity to accurately predict a lead’s potential and optimize sales resources. This approach will lead to better identification of high value leads and improved conversion rates.
Book a CrmOne Demo
Experience the CrmOne simplicity and power. Our experts will show you the best ways to use it and answer your questions in real time. See how CRMOne fits your needs.
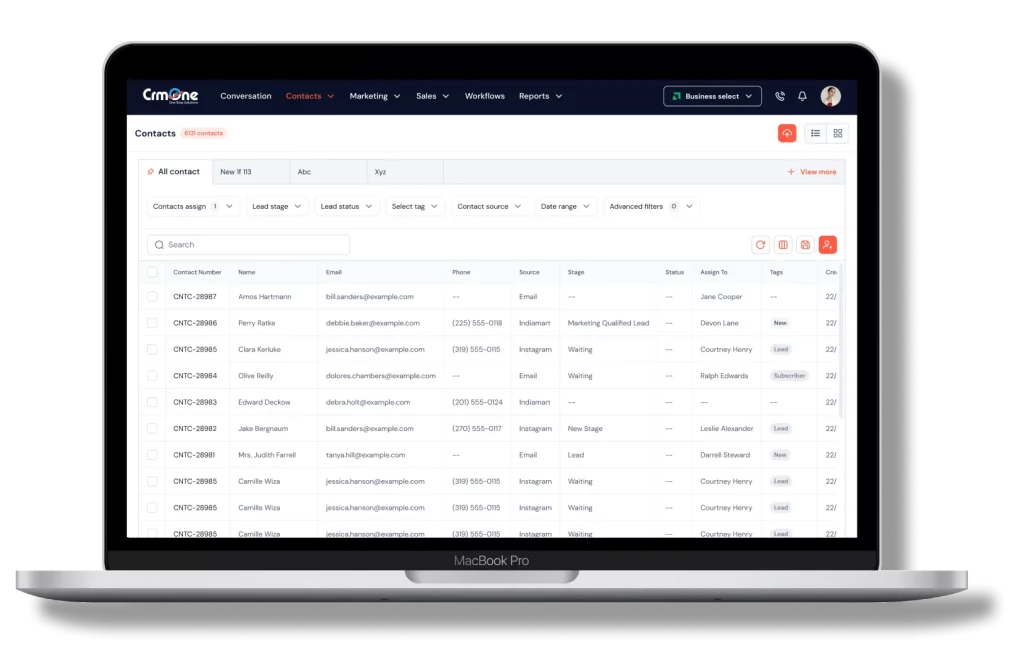
Implementing Predictive Lead Scoring
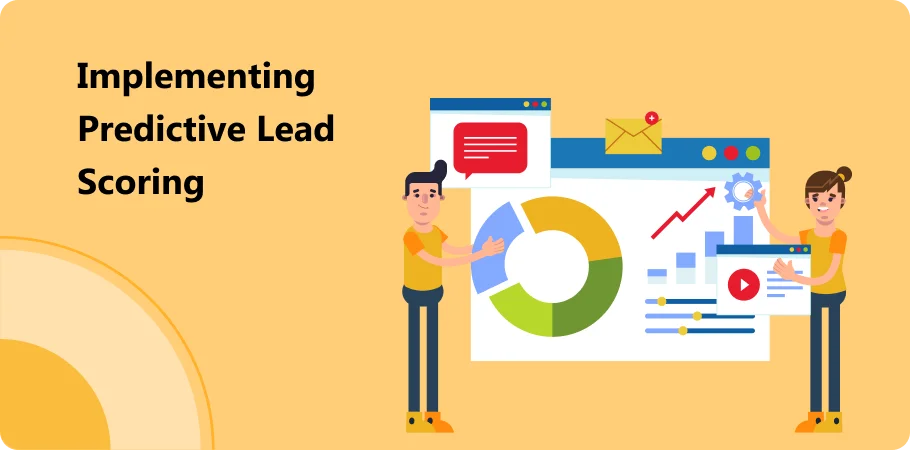
Steps to Develop a Predictive Lead Scoring System
1. Define Objectives:
Clearly outline what you want to achieve with AI powered lead scoring. Identify the main goals and metrics.
2. Collect Data:
Gather all relevant data, including behavioral data, demographic information, and historical and current data.
3. Data Cleaning:
Ensure the data is accurate and clean. Remove any duplicates or irrelevant data points.
4. Select Algorithms:
Choose the right machine learning algorithms that fit your objectives and data type.
5. Build the Model:
Develop the lead scoring model using the selected algorithms. Train the model with historical data to learn patterns.
6. Test the Model:
Validate the model by testing it with a subset of data. Check its accuracy and make necessary adjustments.
7. Implement the Model:
Integrate the model into your CRM or sales system. Start using it to rank potential customers and assign lead scores.
8. Monitor and Refine:
Continuously monitor the model’s performance. Refine and update it regularly to maintain accuracy.
Best Practices for Implementation
Start Small:
Begin with a small, manageable dataset. Expand as you gain confidence in the model.
Involve Stakeholders:
Ensure that both the sales and marketing teams are involved in the process.
Regular Updates:
Continuously update the model with new data to keep it relevant and accurate.
Clear Communication:
Clearly explain the lead scoring process to all team members to ensure everyone understands how it works and its benefits.
Tools and Technologies for Predictive Lead Scoring
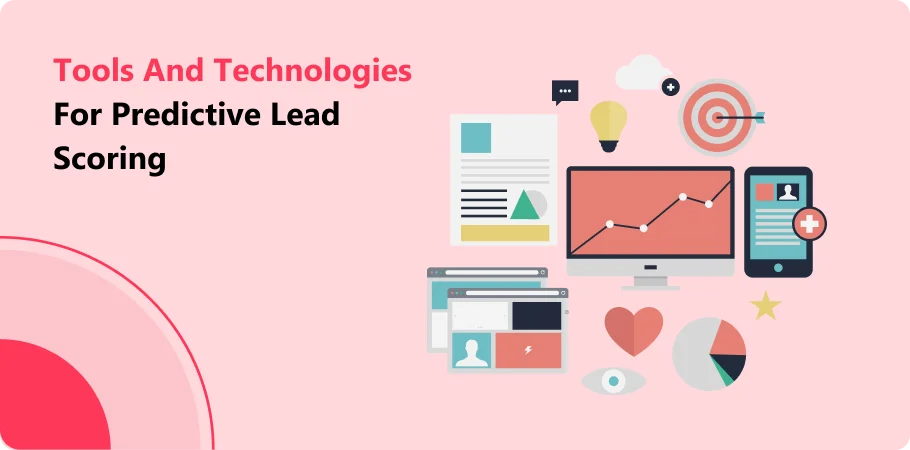
CRMOne is a robust CRM platform designed to enhance your sales processes with its advanced predictive lead scoring capabilities. Here are some of the standout features of CrmOne:
- Advanced Analytics: CRMOne uses machine learning algorithms to analyze historical data and predict future behaviors, ensuring that you focus on leads most likely to convert.
- Customization: Tailor the lead scoring model to fit your specific business requirements. CRMOne allows you to set custom criteria and scoring rules, ensuring the scoring system aligns perfectly with your sales strategy.
- Integration: Seamlessly integrates with other marketing and sales tools, allowing for a unified approach to managing and analyzing leads.
- Real-Time Updates: Stay up-to-date with real-time lead score updates, ensuring your sales team always has the most current information.
- User-Friendly Interface: CrmOne’s intuitive interface makes it easy for your team to adapt and utilize the lead scoring features without extensive training.
- Automation: Automate routine tasks such as follow-up emails and reminders based on lead scores, helping your sales team stay focused on high-priority leads.
- Reporting and Insights: Gain valuable insights through detailed reports and dashboards that highlight key metrics and trends in your lead management process.
How to Choose the Right Technology for Your Needs
Assess Your Needs:
Identify the specific needs of your sales and marketing teams. Determine what features are most important for your business.
Budget:
Consider your budget. Some tools may be more cost-effective for smaller businesses, while others may offer advanced features at a higher cost.
Integration:
Ensure the tool can integrate seamlessly with your existing CRM and other marketing tools.
Integration:
Choose a tool that can scale with your business as it grows.
Ease of Use:
Opt for a user-friendly tool that your team can easily learn and use without extensive training.
In summary, implementing predictive lead scoring involves a series of steps from defining objectives to continuously refining the model. Using the right tools and technologies, like AI powered lead management software, can significantly enhance your ability to rank potential customers and identify qualified leads. This helps in targeting good leads and predicting their future behavior, ultimately improving your sales and marketing efforts.
Challenges and Solutions in Predictive Lead Scoring
Common Obstacles
Data Quality:
Ensuring the accuracy and relevance of data is crucial. Poor data can lead to incorrect lead scores.
Integration Issues:
Integrating predictive lead scoring systems with existing CRM and marketing tools can be complex.
User Adoption:
Getting the sales and marketing teams to trust and use the new system can be challenging.
Resource Allocation:
Predictive lead scoring requires time, money, and technical expertise, which might be limited.
Issues Related to Data, Technology, and Processes
Data Silos:
Data stored in separate systems can be hard to combine and analyze.
Technical Expertise:
Building and maintaining predictive lead scoring systems requires skilled personnel.
Process Changes:
Implementing a new system often requires changes to existing processes, which can meet resistance from team members.
Strategies to Overcome Challenges
Data Quality Management:
Regularly clean and update your data. Use tools and software that ensure data accuracy.
Integration Planning:
Plan the integration process carefully. Choose tools that are compatible with your existing systems.
Training and Support:
Provide thorough training for your team. Offer continuous support to help them understand and trust the new system.
Resource Allocation:
Start small to manage resources effectively. Gradually expand the system as you see positive results.
Tips for Successful Adoption and Optimization
Clear Communication:
Explain the benefits of predictive lead scoring to your team. Show how it can improve lead generation and contribute to business growth.
Incremental Implementation:
Implement the system in stages. This allows you to address issues early and make necessary adjustments.
Continuous Monitoring:
Regularly monitor the system’s performance. Make updates and improvements based on feedback and data analysis.
Focus on Benefits:
Highlight the positive outcomes, such as improved lead scores and better lead generation. This helps in gaining team buy-in and support.
Conclusion
Predictive lead scoring is transforming the way businesses identify and prioritize leads. By leveraging data and machine learning, it provides a more accurate and efficient method for assessing lead quality. This not only enhances collaboration between sales and marketing teams but also significantly boosts conversion rates and marketing ROI.
The benefits of predictive lead scoring are clear. It allows teams to focus on quality leads, make data-driven decisions, and optimize resources. In today’s competitive market, using predictive analytics in lead scoring is no longer a luxury but a necessity for sustained business growth.
If you haven’t yet considered implementing predictive lead scoring models, now is the time. By integrating these advanced techniques into your lead management strategy, you can stay ahead of the competition and drive better results for your business. Take the first step towards more effective lead generation and improved sales outcomes by exploring how predictive analytics can revolutionize your lead scoring process.
Get started for Free
Start for free today. Boost your sales by clicking the Get Started button. With CrmOne, you can manage leads, sales, and customer service all in one place.